Supervised Classification Using Hebbian Linear Neural Network with A Bias Term (Wo)
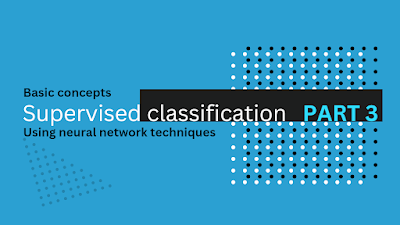
Introduction Hebbian linear neural network with a bias term allows for a translation capability in addition to the rotation that is made using the weights parameters w 1 and w 2. Objectives Familiarize with the concept of supervised classification using linear Neural Network (Hebbian Network) with a bias term. Methodology Using MATLAB code to formulate the learning problem, by computing the net input function (U) using given values of weights and bias term., and derive the Error E, and update weights if applicable until getting the right classification. The result is tested by calculating the target values (t) of the given data. The data distribution and decision line are then plotted. Figure 1 main MATLAB Code Figure 2 main MATLAB Code - Continue Figure 3 MATLAB Code - computation function Observations/Measurements/Data sources Using the following training data set: Table 1 given data set. Pattern No. Feature X 1 Feature X 2 Target (t) 1 0.5 0.1 1 2 0.6 0.2 1 3 0.4 0.3 1 4 1.5 1.9 ...